In today’s data-driven landscape, the integration of machine learning (ML) into data process outsourcing has revolutionized the way organizations manage and analyze their data. As businesses in the USA grapple with large volumes of information, extracting meaningful insights has become a key competitive advantage. Machine learning, coupled with data process outsourcing, offers a powerful solution for deriving actionable insights and driving informed decision-making.
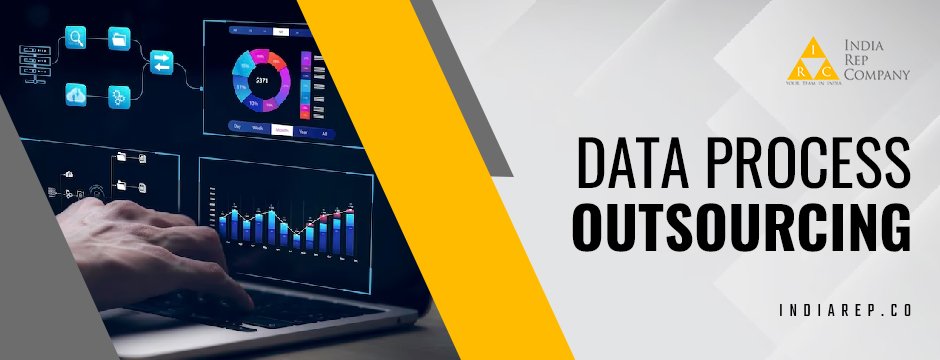
Evolution of Data Process Outsourcing
Traditionally, outsourcing data process primarily focused on managing, organizing, and processing large datasets. However, with the surge in data volume and complexity, businesses in the USA seek more than just data management. They yearn for valuable insights that guide strategic decisions.
Enter machine learning—an advanced technology that enables systems to learn and improve from experience without explicit programming. By integrating ML algorithms into data processing, outsourcing providers can deliver more profound insights, predictive analytics, and personalized solutions to businesses across various industries.
Leveraging Machine Learning for Enhanced Insights
1. Predictive Analytics
Machine learning algorithms analyze historical data patterns, enabling predictive modeling. By recognizing trends and correlations, businesses gain foresight into future outcomes. For instance, in finance, ML predicts market trends or potential risks based on historical market behavior. This predictive power assists businesses in proactive decision-making, anticipating customer demand, optimizing resource allocation, and mitigating potential risks, resulting in a more agile and competitive market stance.
2. Personalized Recommendations
In the context of data process outsourcing, e-commerce and entertainment industries harness ML to offer personalized recommendations. By analyzing user behavior, ML algorithms suggest products, movies, or music that align with individual preferences, enhancing customer satisfaction and engagement. These tailored recommendations not only improve customer experiences but also drive sales, increase customer retention, and foster brand loyalty. Moreover, personalized suggestions based on user habits and preferences serve as a significant factor in influencing purchasing decisions, amplifying overall revenue for businesses.
3. Natural Language Processing (NLP)
NLP, a subset of ML, understands, interprets, and generates human language. In data process outsourcing, NLP aids in sentiment analysis, chatbots, and content categorization, facilitating better customer interactions and content management. Through sentiment analysis, businesses gauge customer feedback, enabling proactive responses to queries or concerns. Chatbots, powered by NLP, offer instant, accurate responses, enhancing customer support experiences and reducing response times. Additionally, content categorization streamlines information management, ensuring relevant content delivery, and optimizing search functionalities for users, ultimately enhancing user satisfaction.
Benefits of ML Integration in Data Process Outsourcing
1. Improved Accuracy and Efficiency
ML algorithms continuously learn and adapt, enhancing accuracy in data processing. As a result, businesses witness improved decision-making based on precise insights generated from cleaned and structured data. This accuracy minimizes errors, providing reliable insights that empower organizations to make data-driven decisions swiftly, thereby bolstering operational efficiency and optimizing resource allocation effectively.
2. Cost Savings
Automating data analysis tasks through ML reduces manual effort and time, leading to cost savings. Outsourcing providers or data entry virtual assistants can offer more competitive pricing while delivering higher value to clients. The reduction in manual labor and operational costs due to ML-driven automation allows for more efficient resource allocation. Additionally, the streamlined processes enable businesses to optimize their budget allocation, redirecting saved costs towards innovation and growth initiatives, thereby fostering a competitive edge in the market.
3. Scalability and Flexibility
ML models can handle vast and varied datasets, allowing scalability without compromising efficiency. Businesses benefit from adaptable solutions tailored to their evolving needs. With ML’s scalable architecture, businesses can effortlessly accommodate expanding datasets, ensuring seamless operations without compromising performance. The flexibility inherent in ML-powered solutions enables quick adaptation to change business requirements, ensuring that the solutions provided through outsourcing are dynamic and responsive to evolving industry landscapes and client demands.
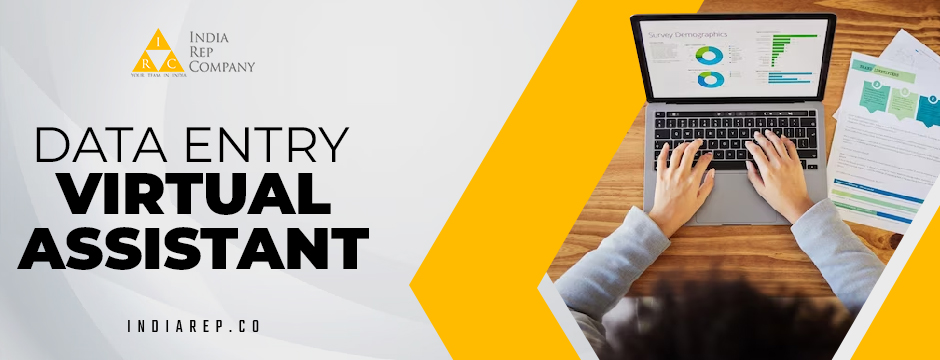
Challenges and Considerations
While ML integration in data process outsourcing offers immense benefits, it also presents challenges:
1. Data Security and Privacy
Handling sensitive data requires robust security measures. Outsourcing providers must ensure compliance with data protection regulations to safeguard client information. Implementing encryption, access controls, and regular security audits becomes imperative to prevent data breaches. Stringent adherence to global standards like GDPR and HIPAA ensures the confidentiality and integrity of sensitive data.
2. Skill Gap
Implementing ML necessitates skilled professionals proficient in algorithm development and model training. Finding and retaining such talent can be challenging. Organizations must invest in continuous upskilling programs, collaborations with educational institutions, and talent acquisition strategies to bridge the gap. Building internal expertise or partnering with specialized firms helps overcome the shortage of skilled ML practitioners.
3. Ethical Concerns
ML algorithms are subject to bias based on training data. Providers must address bias issues to ensure fairness and equity in the insights generated. Employing diverse datasets and rigorous bias detection mechanisms is critical. Regular auditing of algorithms and promoting diversity in development teams aid in minimizing biases, ensuring ethical AI practices, and fostering trust among users and stakeholders.
Future Outlook
As technology evolves, the integration of machine learning in data process outsourcing will continue to evolve. Advancements in AI and ML models will offer more sophisticated capabilities, enhancing insights and decision-making. The future holds promise for AI-driven innovations, such as improved deep learning models, reinforcement learning algorithms, and enhanced neural networks.
These advancements will pave the way for more accurate predictive analytics, advanced pattern recognition, and real-time data processing capabilities. Additionally, the convergence of machine learning with emerging technologies like edge computing and the Internet of Things (IoT) will further revolutionize data processing, enabling faster and more efficient data analysis, ultimately driving innovation and shaping a more data-driven future.
Summary
Machine learning integration in data process outsourcing is revolutionizing the way businesses extract insights from data. By leveraging ML algorithms, outsourcing providers offer accurate, scalable, and actionable insights crucial for strategic decision-making. While challenges exist, the benefits of ML integration far outweigh the hurdles, promising a future where data-driven insights drive innovation and success across industries.
In conclusion, embracing the fusion of machine learning and data process outsourcing empowers businesses to extract maximum value from their data, propelling them toward a more competitive and informed future. If you want to outsource data processing to India for your USA business, choose India Rep Company.